
From Prediction to Agile Interventions in the Social Sciences
Funded by MKW NRW as part of MKW-Profilbildung 2020
The interdisciplinary research area From Prediction to Agile Interventions in the Social Sciences (FAIR) brings together researchers from the data sciences, statistics, education sciences, psychology, rehabilitation studies, and sociology. FAIR researchers from these different disciplines focus on the development and application of innovative research methods from the data sciences and use them to address societal challenges in highly relevant areas such as education, health, and societal inclusiveness and participation.
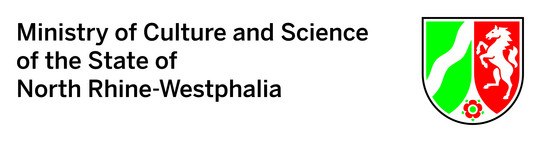
Projective item response theory models for count data and their application as interpretable approximations to black-box machine learning models
Funded by the German Research Foundation (DFG)
Compared to IRT methods for binary data, count data IRT models are underdeveloped. Targeted further method development for count data allows to include unstructured indicators for latent variables, linking IRT more closely to machine learning methods. The research project develops projective IRT models for count data. In particular, projections can be made onto principal dimensions of commonly used existing models. The projective models find use as well-interpretable models in situations where a black-box machine learning model is used for its predictive or classification goodness. This results in approximations to black-box models that help to better understand them. Since multi- and even high-dimensional latent variable constellations are numerically expensive, an EM algorithm for a general count data IRT model is also developed.
Publications
-
Beisemann, M. (2022). A flexible approach to modelling over‐, under‐and equidispersed count data in IRT: The Two‐Parameter Conway–Maxwell–Poisson Model. British Journal of Mathematical and Statistical Psychology. (Advance online publication.) https://doi.org/10.1111/bmsp.12273
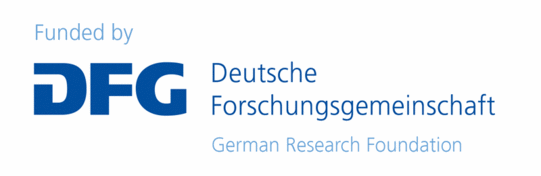